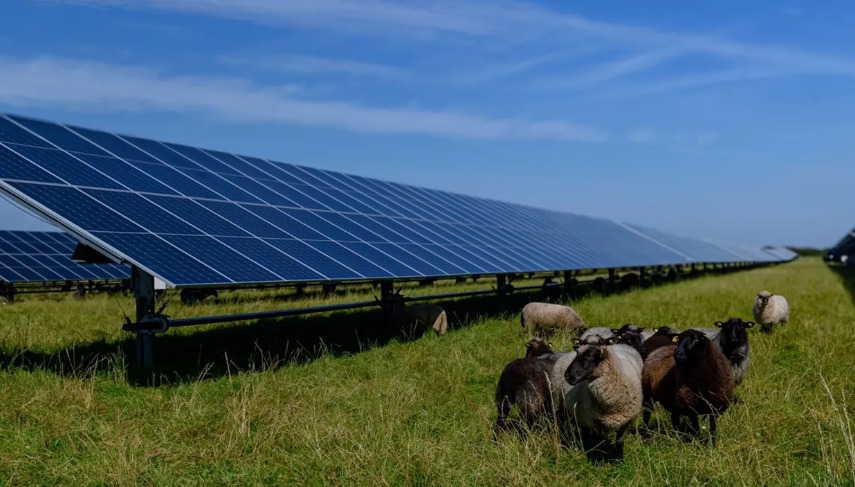
This tool, ‘Photovoltaic Performance Simulator’ is discussed in the study ‘On the Performance Limits of Agrivoltaics—From Thermodynamic to Geo-Meteorological Considerations’, which concludes that organic semiconductor-based components, deployed as part of semitransparent PV installations, have “comparable” performance to inorganic semiconductor-based materials, such as silicon, in agriPV projects.
However, the report notes that there is a wide range of variables that affects the output of agriPV projects, and different types of material will behave differently in a range of environments; “it is never just a matter of maximising transmission and power generation,” according to the report.
Comparing lab results to field results
“Its main aim is to couple what people measure in the lab with what we actually get out in the field,” said Kay, who developed the Photovoltaic Performance Simulator. “It’s got this function where it can get real-world geographical data for a given location, and you can use this then together with a device model to try to figure out how much power you would get out [of a solar module].
“There’s no geographical model in the tool; what it does is speak to the National Solar Radiation Database, (NSRDB) from the National Renewable Energy Laboratory (NREL),” continued Kay, who noted that the tool also uses the World Cities Database, “which has the latitude, longitude and population information of around 45,000 population centres across the globe.”
The programme then compares this location data with information from a number of industry-leading solar material databases, including the 42,000-device Perovskite Database coordinated by T. Jesper Jacobsson and Eva Unger of German research centre Helmholtz-Zentrum Berlin.
“It uses optical transfer matrix modelling to describe the reflection, absorption and transmission of light by the photovoltaics using the refractive index and absorption coefficient of each constituent layer in the device,” explained Kay.
“It uses the absorption of light by the photovoltaics to estimate how much power it generates throughout the year, including the light-diffusing effects of clouds, at what voltage and at what current—which are important considerations that need to be made to then either use that power, store it in a battery or even connect it back to the grid.
Optimisation at a systems level
While the tool has been predominantly tested in the agriPV space, Kay noted that a multi-disciplinary team has worked on the project, including researchers from the University of Oxford and Imperial College London, and this team has gone on to use the tool in other fields.
“At the moment, we’re looking more at indoor photovoltaics, trying to power Internet of Things sensors and things like that,” said Kay. “For that sort of project, we’re adding lots of different light sources: lots of different LED spectra, fluorescent light sources and things like that. We’re trying to look at low-light conditions as well.
When asked about other uses, Kay suggested the tool could be used in the building-integrated photovoltaics (BIPV) space, where a module’s colour is important, as people “will want their buildings to look good”.
“For agriPV, it doesn’t really matter what light is being reflected, but it does matter what light is being transmitted through the device, because if your plants don’t get enough red or blue light, the growth of the photosynthesis will be affected,” said Kay. “That’s something we’ve tried to account for in the tool using optical transfer matrix modelling.”
There is a concern, however, that the needs of multi-use systems, such as agriPV or BIPV, can pull developers in different directions; processes that make a solar panel more efficient are not necessarily the same processes that will encourage plant growth, or aid in the design of a building. When asked about this, Kay expressed optimism that the use of tools such as his, and the most sophisticated collected and management of data in the solar sector more broadly, will help to resolve these challenges.
“Data collection and analysis is more important now than ever,” said Kay. “Not just in terms of selecting photovoltaic materials, but also in terms of running an agriPV system.
“We’re trying to optimise agriPV at a systems level, not just considering the solar panel. That’s the hope with agriPV, you don’t have to have this competition if you do it right, and the two can co-exist. That’s what this analysis is trying to show; if we find a material that absorbs really well in the near-infrared [spectrum], and then transmits a lot in the visible, we’ll be able to have a good agriPV system.”
The news follows growing interest in the PV modelling space, with Tigo Energy launching its Predict+ AI tool earlier this year, to forecast power generation and consumption across a range of endpoints.